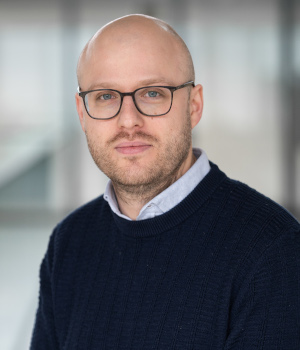
Alex Teytelboym
University of Oxford
Refugees.AI: Improving Refugee Resettlement and Humanitarian Parole
22nd August, 10:15 AM
Abstract
Around 100,000 thousand refugees are resettled to host countries every year. But the current refugee resettlement system is inefficient not only because there are too few resettlement places but also because refugees are resettled to locations where they might not thrive. I will describe Annie MOORE™ , a matching system that seeks to improve employment outcomes of refugees arriving to the United States using dynamic optimization and machine learning. Finally, I will describe how refugees’ preferences are incorporated in RUTH™, a system that is matching Ukrainian refugees to hosting families.Bio
Alex is an Associate Professor at the Department of Economics, University of Oxford, a Tutorial Fellow at St. Catherine’s College, and a Senior Research Fellow at the Institute for New Economic Thinking at the Oxford Martin School. His main research interest is market design, especially the function and design of matching systems, networks, and auctions. He often advises companies, governments and NGOs on these topics. He is giving the Paul Kleindorfer Lecture at the 13th Conference on Economic Design. In 2016, he co-founded of Refugees.AI, an organisation that is developing new technology for refugee resettlement an humanitarian parole.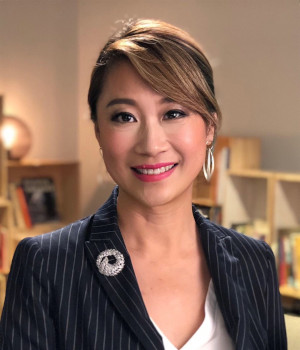
Pascale Fung
Hong Kong University of Science & Technology
Safer Generative ConvAI
22nd August, 2 PM
Abstract
Generative models for Conversational AI are less than a decade old, but they hold great promise for human-machine interactions. Machine responses based on generative models can seem quite fluent and human-like, empathetic and funny, knowledgeable and professional. However, behind the confident voice of generative ConvAI systems, they can also be hallucinating misinformation, giving biased and harmful views, and are still not “safe” enough for many real life applications. The expressive power of generative ConvAI models and their undesirable behavior are two sides of the same coin. How can we harness the fluency, diversity, engagingness of generative ConvAI models while mitigating the downside? Bio
Pascale Fung is a Chair Professor at the Department of Electronic & Computer Engineering at The Hong Kong University of Science & Technology (HKUST), and a visiting professor at the Central Academy of Fine Arts in Beijing. She is an elected Fellow of the Association for the Advancement of Artificial Intelligence (AAAI) for her “significant contributions to the field of conversational AI and to the development of ethical AI principles and algorithms”, an elected Fellow of the Association for Computational Linguistics (ACL) for her “significant contributions towards statistical NLP, comparable corpora, and building intelligent systems that can understand and empathize with humans”. She is an Fellow of the Institute of Electrical and Electronic Engineers (IEEE) for her “contributions to human-machine interactions” and an elected Fellow of the International Speech Communication Association for “fundamental contributions to the interdisciplinary area of spoken language human-machine interactions”. She is the Director of HKUST Centre for AI Research (CAiRE). She is an expert on the Global Future Council, a think tank for the World Economic Forum. She represents HKUST on Partnership on AI to Benefit People and Society. She is a member of the IEEE Working Group to develop an IEEE standard – Recommended Practice for Organizational Governance of Artificial Intelligence. Her research team has won several best and outstanding paper awards at ACL, ACL and NeurIPS workshops.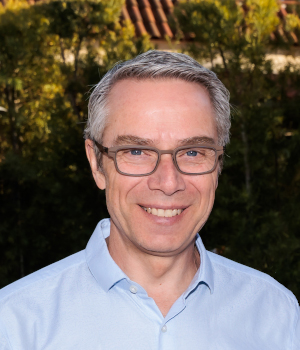
Dieter Fox
University of Washington and NVIDIA
Toward Foundational Robot Manipulation Skills
23rd August, 9 AM
Abstract
The last years have seen astonishing progress in the capabilities of generative AI techniques, particularly in the areas of language and visual understanding. Key to the success of these models is the availability of very large sets of images and text along with models that are able to digest such large datasets. Unfortunately, we have not been able to replicate this success in the context of robotics, where robots still struggle to perform seemingly simple tasks such as manipulating objects in the real world. A crucial reason for this problem is the lack of data suitable to train powerful, general models for robot decision making and control.In this talk, I will describe our ongoing efforts toward developing the models and generating the kind of data that might enable us to train foundational robot manipulation skills. To generate large amounts of demonstration data, we sample many object rearrangement tasks in physically realistic simulation environments, generate high quality solutions for them, and then train perception-driven manipulation skills that can be used in unknown, real-world environments. We believe that such skills could provide the glue between generative AI reasoning and robust execution in the real world, thereby providing robots with the basic capabilities necessary to succeed across a wide range of applications.
Bio
Dieter Fox is Senior Director of Robotics Research at NVIDIA and Professor in the Paul G. Allen School of Computer Science & Engineering at the University of Washington, where he heads the UW Robotics and State Estimation Lab. Dieter obtained his Ph.D. from the University of Bonn, Germany. His research is in robotics and artificial intelligence, with a focus on state estimation and perception applied to problems such as robot manipulation, mapping, and object detection and tracking. He has published more than 200 technical papers and is the co-author of the textbook “Probabilistic Robotics”. He is a Fellow of the IEEE, AAAI, and ACM, and recipient of the 2020 Pioneer in Robotics and Automation Award. Dieter also received several best paper awards at major robotics, AI, and computer vision conferences. He was an editor of the IEEE Transactions on Robotics, program co-chair of the 2008 AAAI Conference on Artificial Intelligence, and program chair of the 2013 Robotics: Science and Systems conference.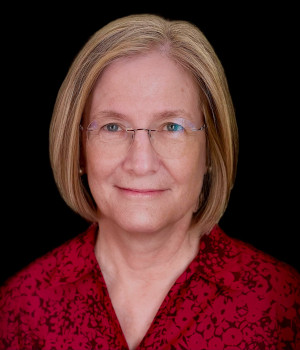
Mary Lou Maher
University of North Carolina at Charlotte
Enhancing Human Creativity Through the Synergy of Cognitive and Connectionist Models
23rd August, 2 PM
Abstract
Creativity is foundational to human learning, social and scientific innovation, quality of life, and economic prosperity and is practiced in context by all of us in our work lives, education, and social interactions. Computational creativity, while inspired by human creativity, lacks the intention and transformative characteristics of human creativity. This presentation proposes that the goal of computational creativity should be to support and enhance human creativity rather than mimic it. Deep learning in large language models demonstrates a kind of computational creativity based on how we use language but lacks the ability to model abstractions of creativity relevant to the intention of humans. Cognitive systems demonstrate creativity through the application of models such as analogy, framing/reframing, novelty, and surprise but lack the ability to generalize beyond their knowledge base. The synergy of cognitive and connectionist models of creativity has the potential to address the limitations of each as computational models while interacting with humans as a co-creative partner.Bio
Dr. Mary Lou Maher is a Professor in the College of Computing and Informatics at UNC Charlotte. She is a co-Director of the Human-Centered Computing Lab. She has held appointments at Carnegie Mellon University, MIT, Columbia University, US National Science Foundation, and the University of Maryland. Her research lies at the intersection of Artificial Intelligence and Human-Computer Interaction. Her recent research areas include AI-based models of novelty and surprise, evaluating ideation in human-AI co-creativity, human-centered AI interaction design, and personalized learning systems that encourage curiosity.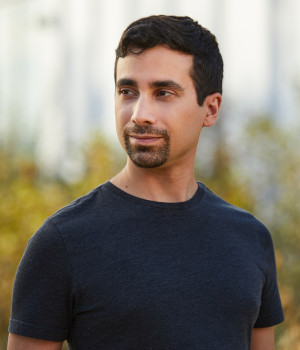
Noam Brown
OpenAI
CICERO: Human-Level Performance in the Game of Diplomacy by Combining Language Models with Strategic Reasoning
24th August, 9 AM
Abstract
In this talk I will describe CICERO, the first AI agent to achieve human-level performance in Diplomacy, a strategy game involving both cooperation and competition that emphasizes natural language negotiation and tactical coordination between seven players. CICERO integrates a language model with planning and reinforcement learning algorithms by inferring players’ beliefs and intentions from its conversations and generating dialogue in pursuit of its plans. Across 40 games of an anonymous online Diplomacy league, CICERO achieved more than double the average score of the human players and ranked in the top 10% of participants who played more than one game.Bio
Noam Brown is an AI researcher investigating multi-agent systems, planning, and negotiation. He co-created Libratus and Pluribus, the first AIs to defeat top humans in two-player no-limit poker and multiplayer no-limit poker, respectively. Noam was also the lead research scientist for CICERO, the first AI to achieve human-level performance in the strategy game Diplomacy. He has received the Marvin Minsky Medal for Outstanding Achievements in AI, was named one of MIT Tech Review’s 35 Innovators Under 35, and his work on Pluribus was named by Science as one of the top 10 scientific breakthroughs of 2019. Noam received his PhD from Carnegie Mellon University, for which he received the AAMAS Victor Lesser Distinguished Dissertation Award, the AAAI ACM-SIGAI Dissertation Award, and the CMU School of Computer Science Distinguished Dissertation Award.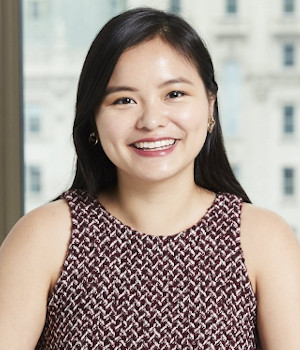
Alice Xiang
Sony
Mirror, Mirror, on the Wall, Who’s the Fairest of Them All?
24th August, 2 PM
Abstract
Debates in AI ethics often hinge on comparisons between AI and humans: which is more beneficial, which is more harmful, which is more biased, the human or the machine? This question, however, is often a red herring. It ignores what is most interesting and important about AI ethics: AI is a mirror. AI reflects patterns in our society, just and unjust, and the worldviews of its human creators, fair or biased. The question then is not which is fairer, the human or the machine, but what can we learn from this reflection of our society and how can we make AI fairer? This talk will discuss three major intervention points—data curation, algorithmic methods, and policies around appropriate use—and how challenges to developing fairer AI in practice stem from this reflective property of AI.Bio
Alice Xiang is the Global Head of AI Ethics at Sony. As the VP leading AI ethics initiatives across Sony Group, she manages the team responsible for conducting AI ethics assessments across Sony’s business units and implementing Sony’s AI Ethics Guidelines. Sony is one of the world’s largest manufacturers of consumer and professional electronics products, the largest video game console company and publisher, and one of the largest music companies and film studios. In addition, as the Lead Research Scientist for AI ethics at Sony AI, Alice leads a lab of AI researchers working on cutting-edge research to enable the development of more responsible AI solutions. Alice also recently served as a General Chair for the ACM Conference on Fairness, Accountability, and Transparency (FAccT), the premier multidisciplinary research conference on these topics. Alice previously served on the leadership team of the Partnership on AI. As the Head of Fairness, Transparency, and Accountability Research, she led a team of interdisciplinary researchers and a portfolio of multi-stakeholder research initiatives. She also served as a Visiting Scholar at Tsinghua University’s Yau Mathematical Sciences Center, where she taught a course on Algorithmic Fairness, Causal Inference, and the Law. She has been quoted in the Wall Street Journal, MIT Tech Review, Fortune, Yahoo Finance, and VentureBeat, among others. She has given guest lectures at the Simons Institute at Berkeley, USC, Harvard, SNU Law School, among other universities. Her research has been published in top machine learning conferences, journals, and law reviews. Alice is both a lawyer and statistician, with experience developing machine learning models and serving as legal counsel for technology companies. Alice holds a Juris Doctor from Yale Law School, a Master’s in Development Economics from Oxford, a Master’s in Statistics from Harvard, and a Bachelor’s in Economics from Harvard.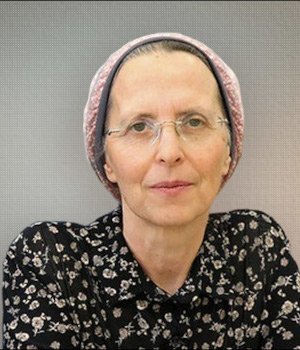
Sarit Kraus
Bar-Ilan University
Human-agent collaboration: facing the challenges of super-intelligent agents.
25th August, 9 AM
Abstract
Software and physical agents’ capabilities, autonomy, and efficiency are experiencing a remarkable surge. However, these advancements pose considerable challenges for humans who must interact, coordinate, or collaborate with these super-intelligent agents. The challenges arise from two primary sources. Firstly, they originate from the human imperative to comprehend and adjust to the behaviors and decision-making of super-intelligent agents, which possess ever-expanding capabilities. Additionally, the complexities inherent in human-agent interactions further contribute to these challenges. Conversely, certain tasks reveal surprising limitations in agents, creating opportunities for people to make valuable contributions to collaborative efforts. One crucial aspect is that these agents may lack a profound comprehension of social and ethical values, potentially leading to catastrophic consequences when attempting to navigate conflicts within efficiency and ethical principles. As a result, it becomes essential for agents to possess the capability to understand and model human decision-making. Additionally, these agents must be able to provide transparent explanations for their decisions and policies, ensuring effective communication with humans. Consequently, humans may deem it essential to convey their preferences and ethical values to the agents, intervening in their actions whenever necessary. During the presentation, I will showcase the challenges that arise when humans are required to collaborate with drones, autonomous vehicles, and negotiating and mediating agents. Moreover, I will explore potential solutions to address these challenges effectively.Bio
Sarit Kraus (Ph.D. Computer Science, Hebrew University, 1989) is a Professor of Computer Science at Bar-Ilan University. Her research is focused on intelligent agents and multi-agent systems integrating machine-learning techniques with optimization and game theory methods. In particular, she studies the development of intelligent agents that can interact proficiently with people and with robots. For her work, she received many prestigious awards. She was awarded the IJCAI Computers and Thought Award, the ACM SIGART Agents Research Award, the ACM Athena Lecturer, the EMET prize and was twice the winner of the IFAAMAS influential paper award. She is an ACM, AAAI and EurAI fellow and a recipient of the advanced ERC grant. She also received a special commendation from the city of Los Angeles and was IJCAI 2019 program chair. She is an elected member of the Israel Academy of Sciences and Humanities.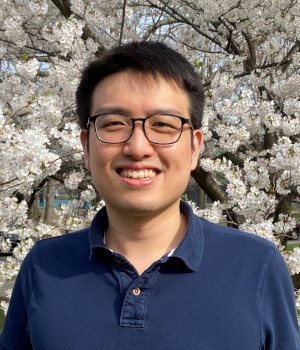
Pin-Yu Chen
IBM, USA.
An Eye for AI: Towards Scientific Approaches for Evaluating and Improving Robustness and Safety of Foundation Models
25th August, 10:15 AM
Abstract
Foundation models, which use deep learning pre-trained on large-scale unlabeled data and then fine-tuned with task-specific supervision, have become a prominent technique in AI technology. While foundation models have great potential to learn general representations and exhibit efficient generalization across domains and data modalities, they can pose unprecedented challenges and significant risks to robustness and safety. This talk outlines recent challenges and advances in the robustness and safety of foundation models. It also introduces the “AI model inspector” framework for comprehensive risk assessment and mitigation, and provides use cases in generative AI and large language models.Bio
Dr. Pin-Yu Chen is a principal research scientist at IBM Thomas J. Watson Research Center, Yorktown Heights, NY, USA. He is also the chief scientist of RPI-IBM AI Research Collaboration and PI of ongoing MIT-IBM Watson AI Lab projects. Dr. Chen received his Ph.D. in electrical engineering and computer science from the University of Michigan, Ann Arbor, USA, in 2016. Dr. Chen’s recent research focuses on adversarial machine learning of neural networks for robustness and safety. His long-term research vision is to build trustworthy machine learning systems. He received the IJCAI Computers and Thoughts Award in 2023. He is a co-author of the book “Adversarial Robustness for Machine Learning”. At IBM Research, he received several research accomplishment awards, including IBM Master Inventor, IBM Corporate Technical Award, and IBM Pat Goldberg Memorial Best Paper. His research contributes to IBM open-source libraries including Adversarial Robustness Toolbox (ART 360) and AI Explainability 360 (AIX 360). He has published more than 50 papers related to trustworthy machine learning at major AI and machine learning conferences, given tutorials at NeurIPS’22, AAAI(’22,’23), IJCAI’21, CVPR(’20,’21,’23), ECCV’20, ICASSP(’20,’22,’23), KDD’19, and Big Data’18, and organized several workshops for adversarial machine learning. He is currently on the editorial board of Transactions on Machine Learning Research and serves as an Area Chair or Senior Program Committee member for NeurIPS, ICML, AAAI, IJCAI, and PAKDD. He received the IEEE GLOBECOM 2010 GOLD Best Paper Award and UAI 2022 Best Paper Runner-Up Award. 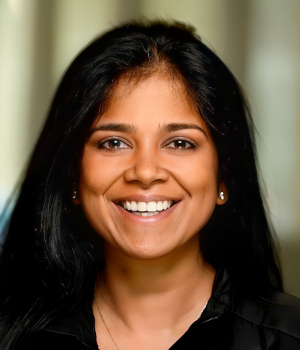
Suchi Saria
Johns Hopkins
AI for augmenting clinical teams: opportunity, technical hurdles, promising results, and open problems
25th August, 2 PM
Abstract
The use of AI in improving medical decision making is one of the most promising avenues for impact. However, turning these ideas into commonly used tools has been significantly harder and slower than predicted. My research has focused on closing fundamental technical gaps related to the development and robust translation of AI-based medical tools from messy, multi-modal observational datasets. My industry experience has given me a first hand view into hurdles that must be tackled for scaling these solutions in the real-world. In 2022, we published 3 manuscripts, featured on the cover of Nature Medicine, that shared results from one of the largest real-world evaluations of a medical AI tool to date. These studies were also the first to show the impact of AI on saving lives. Based on these results, we achieved FDA Breakthrough status. This talk will give an overview on what it takes to go from an idea to a bedside tool. Along the way, I’ll give pointers to new technical ideas and open research problems in AI safety, human-machine teaming, and modeling multi-modal temporal data.Bio
Suchi Saria, PhD, holds the John C. Malone endowed chair and is an associate professor of computer science, statistics, and medicine at Johns Hopkins. She is also is the Founder of Bayesian Health, a leading health AI platform company spun out of her university research. Her methods work has focused on solving challenges in ensuring safe real-world translation of AI in high-stakes applications, multi-modal time series modeling, and causal and counterfactual reasoning for time series data. Her applied research has built on these technical advances to develop novel next generation diagnostic and treatment planning tools that use AI/statistical learning methods to individualize care. Her work has been funded by leading organizations including the NSF, DARPA, FDA, NIH and CDC and featured by the Atlantic, Smithsonian Magazine, Bloomberg News, Wall Street Journal, and PBS NOVA to name a few. She has won several awards for excellence in AI and care delivery. For example, for her academic work, she’s been recognized as IEEE’s “AI’s 10 to Watch”, Sloan Fellow, MIT Tech Review’s “35 Under 35”, National Academy of Medicine’s list of “Emerging Leaders in Health and Medicine”, and DARPA’s Rising Star awardee. For her work in industry bringing AI to healthcare, she’s been recognized as World Economic Forum’s 100 Brilliant Minds Under 40, Rock Health’s “Top 50 in Digital Health”, Modern Healthcare’s Top 25 Innovators, The Armstrong Award for Excellence in Quality and Safety. Her family is from Darjeeling and she loves good tea. Before things got too busy, she did triathlons, drew, and danced. Now she spends her limited free time with her family and traveling going to destinations where she can bike, taste wine, or kitesurf.